Understanding How Artificial Intelligence Works and Its Impact on Our Lives
Artificial Intelligence (AI) has become a hot topic in this digital era. From smartphones to smart homes, AI is increasingly integrated into our daily lives. But, how does Artificial Intelligence work, and what are its impacts for our future? Let’s delve deeper into it.
What is Artificial Intelligence?
Artificial Intelligence, commonly referred to as AI, is a branch of computer science that focuses on creating systems capable of learning from experience, adapting to new inputs, and performing tasks typically requiring human intelligence. In other words, AI is the endeavor to create machines that are ‘intelligent’.
The types of AI
- Rule-Based AI: Using specific rules to generate conclusions.
- Machine Learning: Allowing machines to learn from data.
- Deep Learning: A subset of machine learning that utilizes artificial neural networks with multiple layers.
How Does Artificial Intelligence Work?
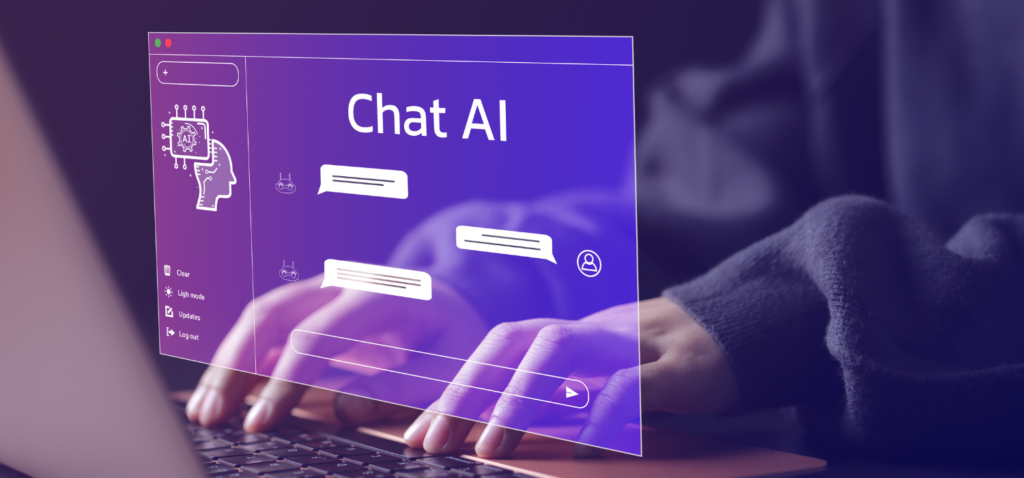
AI works by combining vast amounts of data and smart algorithms. This allows the software to learn from patterns present in the data. Techniques such as machine learning and deep learning are used for data analysis and decision-making.
AI can perform tasks that are typically done by humans, such as understanding speech and recognizing patterns. Its goal is to provide software that can think and explain decisions, akin to human-like interactions with software. AI is used in various industries, from self-driving cars to online shopping recommendations.
In the business sector, AI is often used for automating tasks and analyzing customer data, thereby enhancing operational efficiency. Overall, AI leverages data and intelligent algorithms to make decisions, with the potential for significant impact on society and businesses.
Furthermore, AI works through a series of steps involving data processing and decision-making. The following steps outline how AI operates:
- Input: Data is collected and fed into the AI system, which can take the form of text, images, or speech.
- Processing: The AI system processes the data and determines what to do with it, using algorithms to analyze and identify patterns.
- Output: Based on the processing, the AI system produces outputs that can be used to make decisions.
- Adjustment: If the results are not as desired, the AI system learns from mistakes and may repeat the process in a different way. Algorithmic rules may need to be adjusted to fit the dataset.
- Evaluation: After completing the given tasks, the final step is assessment, where the technology analyzes the data, draws conclusions, and provides necessary feedback before running the algorithms again.
These steps illustrate how AI systems work by combining large datasets with smart algorithms to learn patterns and features within the data, enabling them to perform tasks and make decisions.
Data Collection
A female programmer holding a tablet stands in front of a large screen displaying blue-colored code, highlighting a technology-focused work environment.
It all starts with data. AI relies on data to learn and make predictions or decisions. AI data collection involves mining, organizing, and curating data from various sources to feed AI algorithms.
This process involves gathering and measuring information from various sources, such as numerical, categorical, or free-text data. The collected data is then processed and analyzed to identify patterns and make informed decisions.
The AI system relies on high-quality and relevant data to perform tasks effectively, and the role of data has become increasingly crucial in shaping sophisticated AI models.
The process of data collection is crucial to train AI systems to learn, adapt, and make informed decisions. It involves extensive experience in gathering data from all regions of the world and is a vital process that drives AI systems.
The process of data collection is complex and requires expertise to optimize the potential of AI systems. Therefore, organizations need to ensure the availability and quality of data to successfully implement AI.
Here are some examples of AI data collection methods:
- Crowdsourcing: Involves assigning data collection tasks to the public, providing instructions, and creating a sharing platform.
- In-house data collection: Organizations gather their own data using various methods such as surveys, observations, and interviews.
- Ready-made dataset: Datasets that are already available in the market and can be used for specific AI projects.
- Pengumpulan data otomatis: Memanfaatkan alat dan proses otomatis untuk mengumpulkan dan mengorganisir data dari berbagai sumber.
- Web scraping: Extracting data from websites using automated tools and algorithms.
- Generative AI: Creating synthetic data to complement existing datasets for AI training.
These methods are used to collect, process, and prepare data for training AI models, and each approach has its own advantages and considerations.
Machine Learning Algorithms
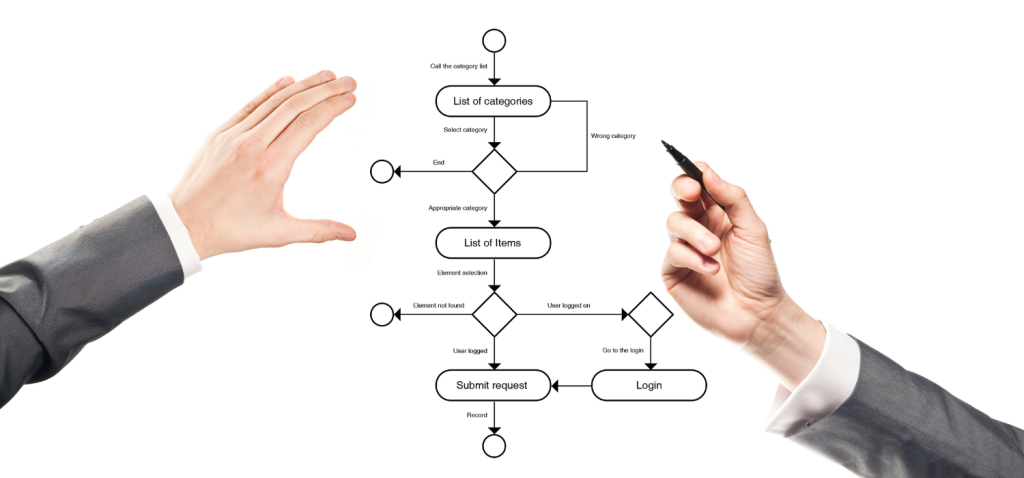
Some popular machine learning algorithms include:
- Linear Regression: Linear regression is an algorithm that establishes a linear relationship between an independent variable and a dependent variable to predict outcomes of future events. It’s a statistical method used in data science and machine learning for predictive analysis. Linear regression is employed for prediction and forecasting tasks, such as sales figures or house prices.
- Logistic Regression: Logistic regression is a supervised machine learning algorithm that addresses binary classification tasks by predicting the probability of outcomes, events, or observations. This model yields binary or dichotomous outcomes limited to two possible results: yes/no, 0/1, or true/false. It’s commonly used for binary classification problems, such as determining whether an email is spam or not.
- Decision Tree: A decision tree is a decision-making model that uses a tree-like structure to represent possible choices and their consequences, such as desired outcomes, costs, and benefits. It’s a clear way to present an algorithm consisting of conditional statements. Real-world examples of decision tree applications include identifying cancerous and non-cancerous cells, as well as providing recommendations to customers regarding car purchases.
- Support Vector Machine (SVM): SVM is a machine learning approach for binary classification problems. Conceptually, this machine maps input vectors nonlinearly to a high-dimensional feature space. Some real-life applications of SVM include face detection, image classification, drug discovery, etc.
- Naive Bayes: Naive Bayes classification is a popular machine learning algorithm used for classification tasks such as text classification. This algorithm belongs to the family of generative learning algorithms, meaning that it models the input distribution for a particular class or category. It is commonly used for text classification, such as spam detection.
- Random Forest: The Random Forest algorithm has gained wide popularity due to its ease of use and adaptability, enabling it to effectively address classification and regression problems. The advantage of this algorithm lies in its ability to handle complex datasets and reduce the risk of overfitting, making it a valuable tool for various predictive tasks in machine learning. Random Forest is a fast algorithm and can efficiently handle missing or erroneous data.
These algorithms are used for various machine learning tasks, such as prediction, classification, and pattern recognition, and are crucial for building machine learning models to solve real-world problems.
Practical applications of AI
From voice recognition to sentiment analysis in social media, AI has found its place in almost every aspect of our lives. AI has various practical applications across different industries. Some examples of AI applications include:
- E-commerce: AI is employed for personalized product recommendations, fraud detection, and chatbots for customer service.
- Healthcare: AI is utilized for medical diagnosis, drug discovery, and personalized treatment plans.
- Manufacturing: AI is employed for quality control, predictive maintenance, and robotics.
- Finance: AI is used for fraud detection, trading, and risk management.
- Gaming: AI is used to create intelligent and human-like Non-Player Characters (NPCs) and enhance the gaming experience.
- Legal Services: AI is employed for legal research, dispute resolution, and contract analysis.
- Agriculture: AI is used for crop monitoring, yield prediction, and harvesting.
READ ALSO: Don’t Miss the Opportunity: How to Use CHAT GPT to Write a Job Application Letter
Examples of AI Applications in Daily Life
Virtual Assistant
Siri, Alexa, and Google Assistant are examples of AI that assist in daily activities by understanding voice commands.
Recommendation System
From Netflix to Spotify, recommendation systems use AI to suggest movies, music, or products based on our preferences.
The Impact of AI on the Future
AI has the potential to revolutionize various sectors, from healthcare to transportation. However, there are also concerns about privacy, ethics, and the replacement of human labor.
Opportunity
High Efficiency: Automation of repetitive tasks enhances efficiency.
Innovation: AI drives innovation in fields such as medicine and renewable energy.
Challenges
Ethical Issues: The use of AI in decision-making raises questions about bias and transparency.
Job Concerns: Accelerated automation can threaten traditional jobs.
Conclusion
Artificial Intelligence is a promising yet challenging breakthrough. By understanding how AI works, we can be better prepared to face the changes it brings and harness its potential for a better future.
Understand how Artificial Intelligence works to stay updated with technological advancements. Looking to expand your network effortlessly? Gotap.id, a provider of digital business cards, offers sophisticated solutions with digital technology to enhance your business interactions. Visit Gotap.id now to start the digital business transformation with innovative and efficient digital business cards!
Ready to upgrade your networking game with GOTAP? Start today and leave a lasting impression at every meeting. Join the future of technology and build your network now!